The Link Between Autism and Sex-Related Neuroanatomy, and Associated Cognition and Gene Expression
Abstract
Objective:
The male preponderance in prevalence of autism is among the most pronounced sex ratios across neurodevelopmental conditions. The authors sought to elucidate the relationship between autism and typical sex-differential neuroanatomy, cognition, and related gene expression.
Methods:
Using a novel deep learning framework trained to predict biological sex based on T1-weighted structural brain images, the authors compared sex prediction model performance across neurotypical and autistic males and females. Multiple large-scale data sets comprising T1-weighted MRI data were employed at four stages of the analysis pipeline: 1) pretraining, with the UK Biobank sample (>10,000 individuals); 2) transfer learning and validation, with the ABIDE data sets (1,412 individuals, 5–56 years of age); 3) test and discovery, with the EU-AIMS/AIMS-2-TRIALS LEAP data set (681 individuals, 6–30 years of age); and 4) specificity, with the NeuroIMAGE and ADHD200 data sets (887 individuals, 7–26 years of age).
Results:
Across both ABIDE and LEAP, features positively predictive of neurotypical males were on average significantly more predictive of autistic males (ABIDE: Cohen’s d=0.48; LEAP: Cohen’s d=1.34). Features positively predictive of neurotypical females were on average significantly less predictive of autistic females (ABIDE: Cohen’s d=1.25; LEAP: Cohen’s d=1.29). These differences in sex prediction accuracy in autism were not observed in individuals with ADHD. In autistic females, the male-shifted neurophenotype was further associated with poorer social sensitivity and emotional face processing while also associated with gene expression patterns of midgestational cell types.
Conclusions:
The results demonstrate an increased resemblance in both autistic male and female individuals’ neuroanatomy with male-characteristic patterns associated with typically sex-differential social cognitive features and related gene expression patterns. The findings hold promise for future research aimed at refining the quest for biological mechanisms underpinning the etiology of autism.
Autism spectrum conditions (henceforth autism) are a set of neurodevelopmental conditions diagnosed four to five times more often in males than in females (1). This male preponderance in prevalence has been attributed partially to an underrecognition of autistic females (2). Even after taking this into account, studies report a male:female ratio of 3:1 (3), implying involvement of neurobiological mechanisms in the sex-biased prevalence of autism. This raises the critical question of how quantitative biological factors related to typical sexual differentiation are involved in the neurobiology of autism. Identifying such mechanisms related to biological sex will broaden our understanding of the sex-differential etiology of autism.
Studies of biological sex differences in the autistic neural phenotype have gained considerable attention in recent years (4). However, how typical sexual differentiation is associated with the neuroanatomy of autistic males and females remains unclear (5–7). Initial efforts show that autistic females tend to exhibit a brain phenotype resembling that of neurotypical males (6,8–11), while findings are more mixed in autistic males (12). It thus remains to be established how a shift toward “neurotypical maleness” differentially informs neurobiological underpinnings in autistic males and females.
Given the neurodevelopmental origins of autism (13), the underlying biological mechanisms are likely to act early ontogenetically. The prenatal period is a critical developmental window in which genetic, hormonal, and neuroimmune organizational factors have long-lasting effects on sexual differentiation and pathways implicated in neurodevelopmental conditions such as autism (14). Different etiological models have been put forward explaining the sex-differential autism likelihood (15–17), for example, the female protective effect (18–20) and increased steroidogenic activity in prenatal neurodevelopment (15, 16, 21, 22). Thus, a key question is how proposed explanations translate into neurobiological cascades from genome to neural and behavioral phenotype. Specifically, we need to better understand how sex-differential neural phenotypes are linked to cognition and genomic mechanisms, and whether they can be traced back to the prenatal developmental period and specific pathways. Recent work shows that studying transcriptional patterns that spatially overlap with brain structural changes provides a mechanistic lens into the physiology of neurodevelopmental conditions (“the transcriptional vulnerability model” [23, 24]). Brain structure is particularly relevant in the developmental biology of neurodevelopmental conditions such as autism (25, 26) and can be considered an intermediate phenotype between genetics and behavior (27). Specifically, autism has been associated with spatially selective alterations in neuroanatomy that have been linked to both autistic behavioral features (28) and genes relevant to these features (29–31).
Motivated by the need to address previous shortcomings, such as small sample sizes (especially of females) and lack of independent replication, tests of methodological robustness (32), and careful accounting for confounding variables (e.g., brain volume [7]), our aim in this study was to comprehensively establish whether the neuroanatomy in male and female autistic people shows, on average, a shift toward (and beyond) the typical male neuroanatomy, and whether this is associated with autism-associated and typically sex-differential cognition and related gene expression. In line with etiological models and evidence suggesting that typical male neurobiology is associated with a higher likelihood of autism (14, 16, 19, 21), we derived three hypotheses, using a T1-weighted structural brain imaging–based sex prediction classifier pretrained in neurotypical males and females to predict male sex: 1) among autistic individuals, females will be less accurately classified than males; 2) among females, autistic individuals will be less accurately classified than neurotypical individuals; and 3) among males, autistic individuals will be more accurately classified than neurotypical individuals. We further examined whether male-shifted neuroanatomy is specific to autism; neurodevelopmentally different across age; associated with specific brain regions and clinical features; and linked to gene expression associated with autism, prenatal development, or sex differences.
To test our hypotheses, we employed a novel deep learning framework leveraging several large-scale neuroimaging data sets with proportionally large numbers of autistic females: the UK Biobank (33), comprising over 10,000 individuals; the Autism Brain Imaging Data Exchange (ABIDE [34, 35]); and the EU-AIMS/AIMS-2-TRIALS Longitudinal European Autism Project (LEAP [36, 37], the largest European multicenter initiative aimed at identifying biomarkers in autism). Based on T1-weighted structural brain images, we first established a sex prediction classifier with high accuracy in the UK Biobank, next transferred and validated it in ABIDE, and then tested it in LEAP to assess sex classification accuracies in autistic males and females. To establish the specificity of our results to autism, we then applied the sex prediction model to an independent sample of individuals with attention deficit hyperactivity disorder (ADHD)—another male-biased neurodevelopmental condition.
We next employed a novel framework to translate global prediction accuracies into spatially specific sex predictions at the level of the brain to identify the most implicated brain regions and associated cognitive features. Finally, to understand associated biological processes, we investigated the enrichment of genes highly expressed in regions associated with sex-differential prediction with a range of (prenatal) genes associated with autism or sex. We tested the hypotheses that genes expressed in male-shifted brain regions are enriched for prenatal genes, autism-associated genes including common genetic variants (38), rare genetic variants (39, 40) and transcriptionally dysregulated genes (41–43), and/or genes differentially expressed by sex or gonadal steroids (44).
Methods
Samples
Pretraining.
We used the preprocessed and quality-controlled first release of UK Biobank data (33, 45), comprising 14,503 individuals (7,584 neurotypical females, 6,919 neurotypical males; ages 44–80 years) to pretrain the convolutional neural networks based on individuals’ T1-weighted structural brain images (46) (see the online supplement).
Validation/transfer.
We selected a sample from the publicly available ABIDE I and II data sets (34, 35). The selection process (see the online supplement) resulted in a total of 1,412 individuals (115 autistic females, 526 autistic males, 239 neurotypical females, 532 neurotypical males; ages 5–56 years) (see information and Table S1 in the online supplement; numbers were balanced across sex and diagnosis at all stages of model training, transfer, and validation).
Testing.
We included participants from the LEAP cohort (36, 37). After selection procedures (see the online supplement), the final sample consisted of 681 individuals (286 autistic males, 109 autistic females, 188 neurotypical males, 98 neurotypical females; ages 6–30 years) (Table 1; see also Figure S1A in the online supplement; numbers were balanced across sex and diagnosis when testing the model). For a list of clinical and cognitive measures included in analyses, see the online supplement.
Variable | Autistic Males (N=286) | Autistic Females (N=109) | Neurotypical Males (N=188) | Neurotypical Females (N=98) | Post Hoc | ||||
---|---|---|---|---|---|---|---|---|---|
Mean | SD | Mean | SD | Mean | SD | Mean | SD | ||
Age (years) | 17.13 | 5.49 | 16.92 | 6.27 | 17.22 | 5.82 | 17.02 | 5.98 | ASC=NT |
IQ | |||||||||
Full-scale IQ | 98.85 | 19.35 | 97.19 | 19.85 | 104.79 | 17.16 | 105.14 | 19.80 | ASC<NT |
Verbal IQ | 97.39 | 19.24 | 97.75 | 19.85 | 103.74 | 18.20 | 105.34 | 20.94 | ASC<NT |
Performance IQ | 100.39 | 21.17 | 96.62 | 21.06 | 105.05 | 19.08 | 103.82 | 19.76 | ASC<NT |
Autism Diagnostic Interview | |||||||||
Social | 17.16 | 6.62 | 15.25 | 6.88 | M>F | ||||
Communication | 13.71 | 5.78 | 12.43 | 5.20 | M>F | ||||
Restricted, repetitive behavior | 4.59 | 2.67 | 3.72 | 2.48 | M>F | ||||
Autism Diagnostic Observation Schedule | |||||||||
CSS | 5.75 | 2.80 | 4.63 | 2.55 | M>F | ||||
Social affect CSS | 6.36 | 2.61 | 5.50 | 2.55 | M>F | ||||
Restricted, repetitive behavior CSS | 4.97 | 2.73 | 4.10 | 2.58 | M>F | ||||
N | % | N | % | N | % | N | % | ||
ADHD | 120 | 42 | 39 | 36 | 13 | 7 | 11 | 11 |
TABLE 1. LEAP sample characterizationa
Specificity.
To assess specificity of results, we selected an independent sample of individuals with ADHD based on the publicly available ADHD200 (47) and the local NeuroIMAGE (48) samples (324 males with ADHD, 130 females with ADHD, 225 neurotypical males, 208 neurotypical females; ages 7–26 years; see information and Table S2 and Figure S1B in the online supplement).
Sex Predictions
The detailed image parameters and steps describing image preprocessing, model training, and testing are outlined in the supplemental information and Table S3 in the online supplement. To summarize, we first pretrained the simple fully convolutional neural network (SFCN) model in UK Biobank for sex classification (i.e., yielding a probability of male sex) using the brain-extracted, bias-corrected, and linearly registered (12 degrees of freedom) T1-weighted images as input (Figure S2 in the online supplement). Next, we applied the pretrained models to ABIDE data via transfer learning (49, 50). For this, we randomly resampled the ABIDE cohort 100 times for training and validation using balanced numbers of individuals in each diagnostic (autism, neurotypical) and sex (male, female) group across all sites (see Figure S1C and S1E and Table S4 in the online supplement). We then applied the 100 fine-tuned models to every individual in LEAP, again using sex- and diagnostic-group-balanced numbers. To summarize prediction results, we computed 1) individual-level ensemble prediction probabilities for every individual based on the median value of the 100 prediction probabilities, which provides an ensemble measure of predictive confidence, and 2) group-level ensemble prediction proportions (i.e., the true positive rate [correctly classified autistic males] and the true negative rate [correctly classified autistic females], which provides an ensemble measure of predictive accuracy, hereafter referred to as “sex prediction accuracy”) for each sex/diagnostic group (autistic male, autistic female, neurotypical male, neurotypical female). For this, all 100 individual-level prediction probabilities were compared against a sex classification threshold, which was defined by the median prediction probability across neurotypical males and females (since we were interested in sex-specific accuracy differences in autism that differed from the neurotypical baseline reference). This implies that the accuracy of the group-level ensemble sex prediction of female and male individuals was the same within the neurotypical group (i.e., the true positive and true negative rates were the same). Finally, group differences in these group-level sex prediction accuracies were computed with one-sample t tests and associated Cohen’s d.
To identify potential age effects, we further checked the sex prediction accuracies as a function of age. For this, we generated four sliding age bins, each spanning 10 years (5–15 years, N=144; 10–20 years, N=204; 15–25 years, N=192; and 20–30 years, N=120), and computed the median sex prediction accuracy for each bin and sex/diagnostic group. To test functional relevance of sex prediction accuracies, we investigated the associations with autism-related, clinical, and sex-differential cognitive features (see the online supplement). The same procedure was also applied to the ADHD sample (see Figure S1D and S1F and Table S4 in the online supplement) and to autistic individuals with and without co-occurring ADHD. Finally, we ran several sensitivity analyses to control for model choice, total brain volume, and age (see the online supplement).
Interpreting Sex Predictions at the Brain Level
Usually, deep learning models can be considered black boxes that do not provide any information on which brain features are most important for classification. Thus, to identify the most predictive and biologically meaningful features that drive our prediction at the brain level, we employed a novel model interrogation approach—region-aligned prediction (RAP) (51)—in the LEAP sample. This method generates spatially resolved estimates of sex prediction accuracy and predicts labels at the brain region level (see the online supplement). Specifically, it aligns the intermediate feature maps (layer “mp4,” corresponding to the 4th layer of the SFCN; see Figure S2 in the online supplement) across all individuals in the data set (including both the training and validation sets) and extracts the feature matrix at one spatial location at a time for each individual. These feature encodings are then used in an L2-regularized multiple regression to predict sex at every spatial location for every individual. Given that the original convolutional neural network was trained 100 times, this resulted in spatially specific sex prediction maps where every spatial location received 100 predictions (one per model) for each individual. We used the median value as the ensemble prediction for each spatial location, resulting in one RAP imaging map per person. Here, each spatial location in each person’s RAP imaging map consists of probabilistic values between 0 and 1 (where 0 means “least likely male” and 1 means “most likely male”). Next, we used these individual RAP imaging maps to compute group-level RAP imaging t-maps (autistic males vs. neurotypical males; autistic females vs. neurotypical females; neurotypical males vs. neurotypical females) to discover the spatial group differences related to the sex predictions. Here, positive t statistics imply a higher male probability in both autistic males and autistic females. Finally, we also applied a quartile split on the prediction probabilities in autistic males and autistic females to also compare highly misclassified and highly correctly classified individuals on their spatial sex predictions.
Cognitive Decoding
We investigated two specific RAP imaging t-maps (autistic females vs. neurotypical females and autistic males vs. neurotypical males) focusing on regions where autistic males and autistic females showed higher male regional predictive probabilities (i.e., positive t values). For this, we used the Neurosynth Image Decoder to visualize the top 100 terms most strongly associated with the two RAP imaging t-maps. To identify regions with the highest male regional predictive probabilities, the RAP imaging t-maps were cluster corrected (voxel-level Z=2.3, p=0.05). Significant regions were then used as masks to extract the average RAP values for each autistic male or female. These region-specific RAP prediction values were then submitted to general linear models to test the association with different cognitive measures. These were picked post hoc based on the cognitive domains implicated by the cognitive decoding analyses (see the online supplement). Results were false discovery rate (FDR) corrected.
Gene Expression Decoding and Enrichment Analyses
To establish genomic associations, we next isolated genes with expression patterns highly similar to the topology present in the RAP imaging t-maps (autistic females vs. neurotypical females and autistic males vs. neurotypical males, with neurotypical females vs. neurotypical males as reference) using the gene expression decoding functionality integrated in Neurosynth (52) and NeuroVault (53). This approach has been used in several autism-related studies (29, 30, 44, 54–56). It uses the six donor brains from the Allen Human Brain gene expression atlas (57) and statistically tests the similarity between spatial gene expression patterns of all 20,787 protein decoding genes and our input maps. For this, an approximate random-effects analysis calculates the slope of best linear fit for each donor. Next, a one-sample t test is performed on the beta estimates to test how consistent the relations between the gene expression and imaging map values are. This way, we identified genes whose spatial expression patterns were highly similar to the evaluated maps, and this consistently across the six donor brains. In the resulting list of genes, only those with expression enriched in brain regions predictive of male phenotypes were retained, that is, genes with positive t statistics resulting from the similarity analysis between positive RAP imaging t-maps (i.e., higher male probability) and high gene expression in these areas (i.e., FDR p<0.05; see the online supplement).
Next, we tested the overlap (i.e., enrichment) of our gene lists with different classes of genes that act prenatally and/or are relevant in the context of autism and sexual differentiation. These included 1) autism-associated genes, including common genetic variants (38), rare genetic variants (39, 40), and transcriptionally dysregulated genes (41–43); 2) genes from prenatal cell types (58); and 3) sex-differentially regulated genes such as genes with sex-differential expression from prenatal brain samples (59, 60) and sex-differentially regulated genes by dihydrotestosterone (DHT) (44, 54, 61) from embryonic neural stem cells, and estrogen (62) (see the online supplement). Analyses examining the enrichment between two lists of genes were implemented using hypergeometric tests based on the sum(dhyper) function in R (44) (see custom code at https://github.com/mvlombardo/utils/blob/master/genelistOverlap.R). The background set size for enrichment analyses was set to the number of protein decoding genes considered in the Neurosynth Gene Expression Decoding analyses (i.e., 20,787). To avoid biasing our findings toward genes expressed in brain (29, 30), we conducted another enrichment analysis using a more conservative list of 16,906 background genes based on real estimates of genes expressed in cortical tissue (63). Enrichment analyses yielded enrichment odds ratios, hypergeometric p values, and FDR q values. Only genes surviving FDR p<0.05 were considered.
Results
Sex Prediction Accuracies in Autism
In ABIDE, at the group level, the proportion of correctly classified autistic females (72%) was on average 9% lower than that of autistic males (82%) (hypothesis 1: Cohen’s d=1.19, p=1.1e−20) and 7% lower than that of neurotypical individuals (79%) (hypothesis 2: d=1.25, p=3.9e−22). The proportion of correctly classified autistic males was 3% higher than that of neurotypical individuals (hypothesis 3: d=0.48, p=1.0e−5) (Figure 1A). These results replicated when testing the 100 ABIDE fine-tuned models in LEAP (Table 2). Specifically, for the same group comparisons, we found that at the group level the proportion of correctly classified autistic females (74%) was on average 12% lower than that of autistic males (86%) (hypothesis 1: d=1.59, p=5.5e−29) and 6% lower than that of neurotypical individuals (80%) (hypothesis 2: d=1.29, p=1.2e−22). The proportion of correctly classified autistic males was 6% higher than that of neurotypical individuals (hypothesis 3: d=1.34, p=1.1e−23) (Figure 1B). These results were also reflected in differences in individual-level predictive confidence in LEAP (Figure 1C): after ranking the individual-level ensemble sex prediction probabilities, we compared the top 10% values using a Wilcoxon rank sum test. Autistic females showed lower predictive confidence (75%) than neurotypical individuals (84%) (W=2.69, p=7.1e−03), while autistic males showed higher predictive confidence (89%) than neurotypical individuals (84%; W=2.08, p=3.8e−02). Effect sizes of sex differences in sex prediction accuracies between autistic and neurotypical individuals were largest in younger individuals, and sex prediction accuracies improved toward adulthood across all groups (Figure 1D). Finally, autistic females showed an association between poorer performance on a mentalizing test with typical sex-differential performance (the “Reading the Mind in the Eyes Test” [64]) and higher individual-level male predictive confidence (t=−2.36, p=0.01, q=0.04; see information and Figure S3A in the online supplement).
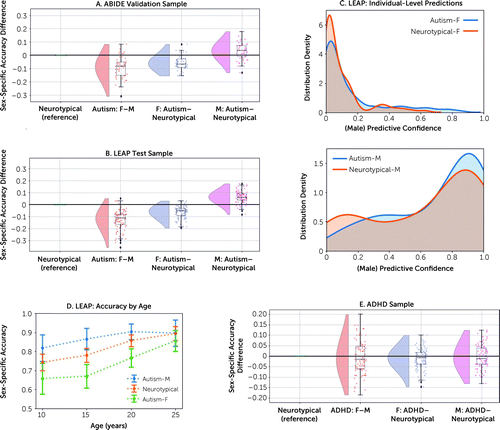
FIGURE 1. Comparison of sex prediction model performance across diagnostic and sex groupsa
aWe compared sex prediction accuracy differences between autistic males and females, autistic females and neurotypical females, and autistic males and neurotypical males in both the ABIDE validation sample (panel A) and the LEAP test sample (panel B). Each dot represents one model out of 100 models in total. Negative values mean that the model performs worse in the first sex/diagnostic group. Panel C shows the distribution of individual-level sex prediction accuracies (i.e., the predictive confidence of being classified as male) across autistic females and neurotypical females in the upper panel and across autistic males and neurotypical males in the lower panel in the LEAP sample. In panel D, we generated four sliding age bins, each spanning 10 years (5–15 years, N=144; 10–20 years, N=204; 15–25 years, N=192; 20–30 years, N=120), and compared the sex prediction accuracies as a function of increasing age. Panel E shows a comparison of sex prediction accuracy differences between males and females with attention deficit hyperactivity disorder (ADHD), females with ADHD and neurotypical females, and males with ADHD and neurotypical males in the combined ADHD200 and NeuroIMAGE sample. F=female; M=male.
Sex-Specific Accuracy | ||
---|---|---|
Cohort and Group | Mean | SD |
ABIDE | ||
Neurotypical | 0.789 | 0.030 |
Autistic females | 0.724 | 0.046 |
Autistic males | 0.818 | 0.050 |
LEAP | ||
Neurotypical | 0.800 | 0.024 |
Autistic females | 0.737 | 0.053 |
Autistic males | 0.863 | 0.041 |
ADHD | ||
Neurotypical | 0.733 | 0.046 |
ADHD females | 0.722 | 0.069 |
ADHD males | 0.730 | 0.064 |
TABLE 2. Sex-specific prediction accuracies across the autism and ADHD samplesa
In the ADHD sample, sex prediction accuracies did not differ across the groups (Figure 1E, Table 2; see also information and Figure S4 and Table S5 in the online supplement). Also, in LEAP, the patterns remained the same when autistic individuals were stratified according to co-occurring ADHD (see Figure S5 and Table S6 in the online supplement). All sets of control analyses confirmed these observed patterns (see Figure S6 and Table S7 in the online supplement).
Region-Specific Sex Predictions
The most differentiating regions between neurotypical males and neurotypical females were in posterior auditory cortex and frontal-temporal/-occipital white matter pathways (Table S8 in the online supplement and Figure 2A). Similarly, the regions with the highest shifts toward maleness in autistic females were also in posterior auditory cortex regions, cerebellum, and occipital fusiform gyrus (gray matter) and corpus callosum and frontal-temporal/-occipital white matter pathways (Figure 2B; see also Table S9 in the online supplement). In autistic males, regions most similar to neurotypical males were mostly in subcortical regions (amygdala, pallidum, thalamus), cerebellum, frontal medial cortex, and supplementary motor cortex (gray matter) and in superior fronto-occipital fasciculus (white matter) (Figure 2C; see also Table S10 in the online supplement). The neurotypical males–versus–neurotypical females RAP imaging t-map was correlated with the autistic females–versus–neurotypical females RAP imaging t-maps at r=0.38, whereas it was correlated with the autistic males–versus–neurotypical males RAP imaging t-maps at r=0.19, further highlighting the similarity between autistic females and neurotypical males. For differences across highly misclassified and correctly classified autistic females and autistic males, see the information and Figure S7 in the online supplement.
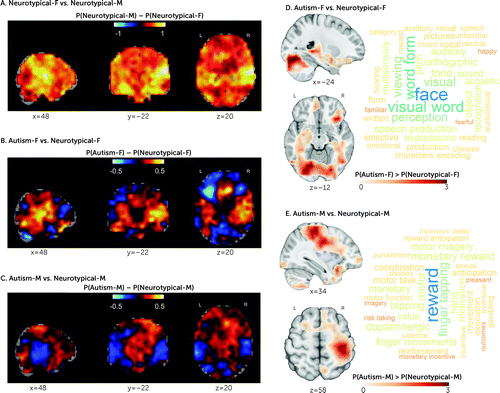
FIGURE 2. Spatial representation of the sex predictions, in region-aligned prediction (RAP) mapsa
aBrain maps in panels A–C depict Cohen’s d maps associated with the different RAP imaging t-maps (spatial representation of the sex predictions) across neurotypical females versus neurotypical males, autistic females versus neurotypical females, and autistic males versus neurotypical males. The color map encodes the average maleness probability (P; yellow/positive values=higher probability of being classified as male; blue/negative values=higher probability of being classified as female). Panel D shows regions where autistic females showed higher male prediction probabilities than neurotypical females, and panel E regions where autistic males showed higher male prediction probabilities than neurotypical males. To explore the cognitive domains implicated in these (panel D and E), we used the Neurosynth Image Decoder (http://neurosynth.org/decode/) to visualize the top 100 terms most strongly associated with the two RAP imaging t-maps showing correlations with the imaging maps between r values of 0.2 and 0.06. Results showed that the most common cognitive terms associated with the female RAP t-maps were primarily related to face perception, visual processing, and speech in autistic females, and to motor and reward processing in autistic males. F=female; M=male; P=probability; P(Neurotypical-F)=probability of neurotypical females being classified as males; P(Neurotypical-M)=probability of neurotypical males being classified as males; P(Autism-F)=probability of autistic females being classified as males; P(Autism-M)=probability of autistic males being classified as males.
Cognitive Decoding
The most common cognitive terms associated with male-shifted regions in autistic females were primarily related to face perception, visual processing, and speech (Figure 2D), whereas in autistic males, they were primarily related to motor and reward processing (Figure 2E). Based on these results, we tested the association between the region-of-interest-specific RAP-based sex prediction values and cognitive measures in LEAP associated with 1) face processing and communication in autistic females and 2) motor and reward processing in autistic males (see the online supplement for specific measures). While we found no relationships in autistic males, in autistic females there was a significant association between predicted maleness and lower accuracy on the Karolinska Directed Emotional Faces task (t=−2.6, p=0.01, q=0.02) (see Figure S3B in the online supplement).
Gene Expression Decoding and Enrichment Analysis
Across both autistic females and autistic males, genes that correlated with male-shifted regions showed significant enrichment for a set of transcriptionally upregulated genes associated with autism (Figure 3B, leftmost and middle panels). In contrast, genes associated with sex-differential regions between neurotypical males and neurotypical females showed significant enrichment for transcriptionally downregulated autism-associated genes (Figure 3B, rightmost panel). Genes correlated with male-shifted regions in autistic females were significantly enriched for prenatal excitatory neuronal cell types (58) (Figure 3A, middle panel) and with autosomal female differentially expressed genes, X-chromosomal male differentially expressed genes, and genes downregulated by estrogen (Figure 3C, middle panel). Strikingly, transcriptional patterns similar to sex-differential regions in neurotypical males and neurotypical females were similar to male-shifted regions in autistic females (Figure 3A–C, middle and rightmost panels). On the other hand, male-shifted regions in autistic males were significantly enriched for prenatal microglial, progenitor, and radial glial cell types (58) (Figure 3A, leftmost panel) and genes upregulated by DHT and estrogen (Figure 3C, leftmost panel). These results remained largely unchanged when we used a more restrictive background total of 16,906 genes (63) (expressed in cortical tissue) (see Figure S8 in the online supplement) (29, 30).
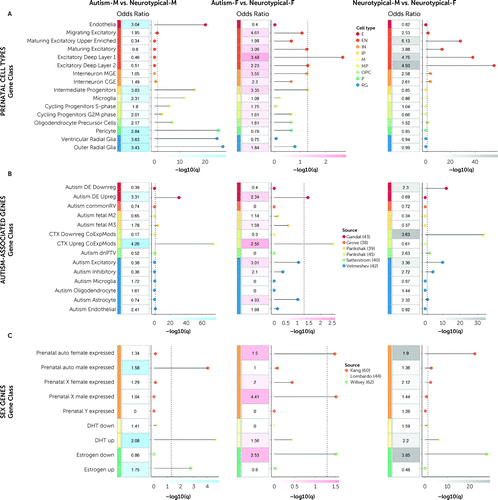
FIGURE 3. Gene set enrichment analysesa
aThe figure shows odds ratios at a false discovery rate–corrected p<0.05 resulting from the gene set enrichment analyses for RAP imaging t-maps (autistic males vs. neurotypical males; autistic females vs. neurotypical females; neurotypical males vs. neurotypical females) and associated gene lists with different classes of genes acting prenatally and relevant in the context of autism and sexual differentiation. Panel A lists genes from prenatal cell types (58): endothelia (E), excitatory neurons (EN; migrating excitatory, maturing excitatory upper enriched, maturing excitatory, excitatory deep layer 1, excitatory deep layer 2), interneurons (IN; interneuron MGE and interneuron CGE), intermediate progenitors (IP), microglia (M), mitotic progenitors (MP; cycling progenitors S-phase and cycling progenitors G2M phase), oligodendrocyte precursors (OPC), pericytes (P), and radial glia (RG; ventricular radial glia and outer radial glia). Panel B lists autism-associated genes, including common genetic variants (Autism commonRV) (38), de novo mutations (fetal gene coexpression modules [Autism fetal M2, Autism fetal M3] [39]; 102 rare, de novo protein truncating genes [Autism dnPTV]) (40), and transcriptionally dysregulated genes (differentially expressed downregulated [Autism DE Downreg], differentially expressed upregulated [Autism DE Upreg] (43); cortical downregulated coexpression modules [CTX Downreg CoExpMods], cortical upregulated coexpression modules [CTX Upreg CoExpMods] [41]; Autism Excitatory, Autism Inhibitory, Autism Microglia, Autism Oligodendrocyte, Autism Astrocyte, Autism Endothelial [42]). Panel C lists sex-differentially expressed genes acting prenatally, such as genes differentially regulated by dihydrotestosterone (DHT) (44, 54, 61), estrogen (62), and (autosomal and X-/Y-chromosome linked) sex-differential gene array expression data from prenatal samples (59, 60). F=female; M=male.
Discussion
Our study demonstrates the overlap of neuroanatomical features characteristic of neurotypical males with those of autistic individuals. This pattern was specific to autism and was not observed in ADHD, pointing to possible different underlying biology in different neurodevelopmental conditions, despite both having a male-predominant prevalence. Overall, autistic females constituted the more critical test case for our hypotheses, likely because masculinizing sex-differentiation effects are less likely to reach a ceiling in females. Consistent with this, we observed an association between greater shifts toward neuroanatomical maleness and cognitive functional difficulties in social sensitivity and emotional face processing in autistic females. Genes relevant to brain regions predictive of male sex in autistic females were highly similar to those differentiating neurotypical males from neurotypical females, primarily comprising prenatal cell types (excitatory neurons) and upregulated autism-associated genes. The findings provide key insights into potential neurobiological and genomic underpinnings associated with male-biased autism prevalence.
We emphasize that the majority of autistic females were correctly classified by sex, and we cannot derive individual-level predictions of autism given the overlapping distributions across neurotypical and autistic individuals. This highlights the large heterogeneity inherent to autism and the importance of identifying biologically meaningful subgroups (65, 66). Among these, at least one subgroup is characterized by multivariate features in brain structure that are more similar to those of neurotypical males. These multivariate results of male-shifted whole-brain patterns add to previous mass-univariate neuroimaging studies that identify shifts toward the neurotypical male profile in autistic females across both brain structure (6, 8, 67) and function (10, 11, 32). A male-like profile in autistic individuals has previously been reported in specific aspects of cognitive style (68,69). Our study extends this observation to multivariate, male-shifted characteristics in brain structure. Importantly, the findings are in line with the notion that there is no strict sexual dimorphism in human neuroanatomy (70, 71), but brains exhibit a “multimorphic” mosaic of male-like and female-like features (72, 73) that can reliably distinguish males from females with above-chance to high accuracy (72, 74, 75). This means that not all autistic individuals have an “extreme male brain,” but multivariate patterns characteristic of neurotypical males are on average more common in a subset of autistic individuals who are overall shifted toward the male neurophenotype. We previously reported such functional brain mosaicism in autistic males who exhibited both shifts toward male- and female-like network connectivity depending on the neural circuit involved (12). We thus emphasize the need for future studies to also examine the shifts toward a female-like neurophenotype in autism (76).
One striking finding is the similarity of neuroanatomical and associated transcriptional patterns between male-shifted regions in autistic females and regions differentiating neurotypical males from neurotypical females. Regions most strongly differentiating neurotypical males from neurotypical females overlapped with well-established sex-differential regions in posterior auditory and visual regions and hippocampus (77, 78). These visual, face, and language processing areas were also among those that least accurately predicted female sex in autistic females and have previously been associated with male-shifted patterns in autistic females (6, 32, 79) as well as with atypical brain structure in autism (80, 81). Higher predicted maleness was also associated with cognitive difficulties in autistic females such as poorer mentalizing and emotional face processing, pointing to the clinical relevance of a male-shifted neurophenotype in autistic females (16, 68). Furthermore, both sex-differential and the cell-type-specific expression patterns overlapping with male-shifted regions in autistic females and with regions differentiating neurotypical males from neurotypical females were highly similar to each other. These multilevel findings jointly suggest that cellular mechanisms mediating neurotypical brain sexual differentiation likely play a role in the etiology of autism, especially in females.
In autistic males, on the other hand, the male-shifted regions were particularly relevant to reward processing and motor functions. The former has been implicated in recent work showing striatal hypoactivation during reward processing in autism (82) and male-specific atypicality in reward learning in an animal model of autism (83). Also, somatomotor networks have frequently been found to be atypical in autism (55, 84–86), and bilateral volumetric increases in somatosensory, motor, and premotor cortex have been associated with fetal testosterone exposure (87).
Particularly, the influence of prenatal masculinization via androgens has been suggested to play a mechanistic role in the etiology of autism (15, 16, 61, 76, 88, 89). Other steroid hormones, including estrogens, have also been found to be associated with autism-related outcomes, when cohorts were restricted to males (21, 22, 90). Also, neuroimaging research shows that gray matter volumes in posterior auditory regions (exhibiting male shifts in autistic females here) and in somatomotor areas (exhibiting male shifts in autistic males here) are associated with increased androgen sensitivity (87, 91).
We further observe that genes relevant to male-shifted regions in autistic males are enriched both for genetic markers of intermediate progenitor (IP) and radial glial (RG) cell types, as well as genes upregulated by DHT and estrogen. In line with this, it has been shown that DHT increases proliferation of IP and RG cell types (92). Also, differences in proliferative processes through symmetric cell division in IP and RG cell types lead to atypical cortical expansion of surface area in autism (56, 93, 94). These downstream consequences of DHT may impact excitatory neuronal signaling, due to expansion of surface area via increased numbers of cortical columns and neurons within each column. Furthermore, genes involved in excitatory postsynaptic potentials and autism-associated genes affecting excitatory neuronal lineages are dysregulated by DHT (44, 54), and consequently, excitation-inhibition imbalance is asymmetrically more affected in autistic males (54).
On the other hand, in autistic females, male-shifted regions are particularly enriched for later differentiated excitatory neurons and genes downregulated by estrogen, but not for genes upregulated by DHT (as in autistic males). This is in line with clinical findings indicating that autistic females may be characterized by a relative imbalance between androgens and estrogens, rather than high steroid levels across all pathways (95, 96). Conditions related to androgen/estrogen imbalances in females (e.g., polycystic ovary syndrome) have previously been linked to autism likelihood in both mothers and their children (97–100). Further, estrogen-regulated signaling can influence region-specific neurodevelopmental male shifts and affect neuronal excitation (101–103), but more studies are needed to demonstrate this in human-derived cell models. Thus, the enrichment effects in IP, RG, and DHT in autistic males and in excitatory neuronal cell types and estrogen in autistic females may be relevant to the overall idea that one key emergent phenomenon of brain masculinization is the effect it has on excitation-inhibition imbalance (104), with potentially different underlying sex-steroid-related mechanisms in autistic males and females (90).
Furthermore, microglial neuroimmunological processes may be major contributors to brain masculinization (14). Werling et al. (59) reported enrichment of male-biased microglial expression with autism-upregulated coexpression modules along with enrichment of female-biased synaptic expression with autism-downregulated coexpression modules, corroborating that molecular downstream pathways regulating neurotypical male development interact with those of autism-associated genes. These observations are consistent with our discoveries that 1) genes expressed in regions overlapping with male-shifted regions in both autistic males and autistic females are enriched for upregulated autism-associated genes mapping onto inflammatory pathways (41) (likely underlying vulnerability mechanisms associated with males [105]), 2) regions differentiating neurotypical females from neurotypical males are enriched for autism-downregulated expression modules (likely underlying protective mechanisms associated with females [105]), 3) the male-shifted regions in autistic males are enriched for prenatal microglial cell types, and 4) downstream transcriptionally dysregulated pathways are more involved in sex-differential processes than they are in upstream genetic susceptibility mechanisms.
Our findings of reduced and superior sex prediction performance in autistic females and males, respectively, are most pronounced in childhood and decrease throughout development to young adulthood. In the age bin of 20–30 years, we do not observe classification differences across groups. Similarly, two other studies in adult samples with mean ages of 22 years (7) and 26 years (5) also showed no sex prediction accuracy differences between autistic and neurotypical adults. It is likely that these age-related patterns are influenced by dynamically unfolding interactions between sex-differential and neurodevelopmental process across the lifespan. Sex differentiation emerges in utero, and many on-average sex differences persist into adulthood, while others are transient and context dependent, meaning they become apparent at specific developmental stages depending on factors such as hormonal milieu and experiences (71). For example, sex steroid hormones are thought to initially act organizationally during perinatal development, and then activationally during puberty to influence the formation and expression of sex differences at different developmental windows (106). Clinically, the rate of pubertal differentiation was, for example, found to interact with the association of autistic traits with fetal testosterone levels in a longitudinal cohort (107). Also, different molecular mechanisms are highly region specific, and they are again expressed at different developmental periods (71). One example of an age-dependent neurophenotype in autism is early brain overgrowth, which is more likely observed in autistic children but not later in life (108, 109). Atypical age-related cortical development has also been demonstrated by deviations from the typical developmental trajectories both in cortical thinning in autistic children and adolescents in longitudinal studies (110–112) and in neuronal differentiation in in vitro models (113). Here, we identified associations with prenatal cell types that have been linked to atypical cortical expansion in autism (56) and likely link back to sex-specific excitation-inhibition imbalance across neurodevelopment. On top of these sex-related mechanisms, gendered experiences, gender role expectations, and gender socialization based on one’s sex assigned at birth in a society may also influence neurobiology and postnatal brain development (2, 114). Considering this mechanistic complexity, future studies (especially longitudinal ones) need to unravel the observed spatiotemporal sex-differential profiles in autism and establish how experiential, environmental, genetic, and hormonal effects interact to differentially contribute to the observed patterns across different developmental windows (e.g., fetal/neonatal stage, childhood, puberty/adolescence, adulthood/reproductive age, and old-age/postmenopause). Finally, we cannot exclude nosological issues and ascertainment bias contributing to the observed age-related patterns. Autism diagnostic practices have been described as male biased (3). It is thus possible that females presenting with “typical/classical” (i.e., male-like) autistic features (which might be related to the observed neuroanatomical patterns) may be diagnosed earlier in life than females presenting with more nuanced phenotypes that are less well captured by current diagnostic practices (115), hence contributing to the age differences in classification performance. We have no available data on age at diagnosis in the present study; however, future studies should disentangle the effects of chronological age and timing of autism diagnosis.
Finally, it has been suggested that the male preponderance in neurodevelopmental conditions may be driven by similar genetic and neuroendocrinological mechanisms. Here, we show that autism is associated with a different sex-differential neuroanatomical profile in males and females than is ADHD. A proposed pathway through which sex-differential prevalence and presentation in ADHD is mediated may be atypical dopaminergic system function modulated by gonadal hormones (116, 117), such as an increase in the striatal dopamine receptor density in prepubertal development in males but not females (118). Future research needs to pinpoint which exact sex-related mechanisms shape differential neurophenotypes in different male-biased conditions.
Strengths and Limitations
Our analytical approach has multiple strengths. We employ four of the largest cohorts available for our populations of interest (neurotypical, autism, ADHD), thus ensuring both replicability and specificity of findings. We carefully address potential confounders, confirming that the results are not driven by differences in brain volume, age, and model choice. Our analyses do not rely on artificial features, but by employing a novel deep convolutional neural network model with superior performance (46), we take whole-brain anatomical data as predictive features. Nevertheless, there are several limitations. Even though our samples span wide age ranges and the results imply the likely involvement of neurodevelopmental mechanisms, only when investigating a longitudinal cohort will we be able to make causal inferences. Further, we did not address social, cultural, or experiential factors (114) that can influence sex-differential brain development (71), or gender identity, which might differ from sex assigned at birth, especially in autistic individuals (119). The data sources employed in the current study did not gather information specifically distinguishing sex at birth and gender identity. As such, the potential for misspecification of participants’ sex at birth exists, but the rate is anticipated to be low. Within this limitation, given that our models were trained to predict biological sex, we used the term sex-specific prediction accuracy. In addition, while utilizing a highly accurate approach for sex classification, the high level of nonlinearity intrinsic to the convolutional neural network model makes straightforward interpretations more difficult compared to simpler linear models. We address this issue by a novel approach for model introspection by means of region-aligned prediction. This approach has the advantage of being able to generate spatially resolved estimates of sex-specific accuracy prediction, but due to the convolutional structure of our model, it achieves this with lower spatial fidelity than the original input data. Yet, being able to spatially resolve the sex prediction accuracy enables the further association analyses with cognition and gene expression. We further note that our enrichment analyses are a correlational approach that cannot reveal the causal genetic mechanisms of our brain structural findings. However, this approach can highlight genes for further molecular analyses to establish mechanisms and directionality of findings (23). Also, we only tested a select number of genes for which there is a prior literature implicating them as robust markers of autism likelihood, prenatal development, and sex-differential biology. While we also acknowledge the methodological limitation of using adult brain expression data in association with prenatal cell types, our results provide important signals that neuroanatomical male shifts in autism are associated with genes that are predominantly expressed in prenatal development and may therefore affect neurodevelopmental processes. Also, the Allen Human Brain gene expression atlas is the most comprehensive and highly sampled gene expression atlas currently available—albeit one based on adult donors only. Once high-resolution, whole-brain, and age-specific gene expression maps become available, future studies should investigate gene expression profiles in the developing human brain. This will further elucidate the genes associated with autism-related sexual differentiation acting at different developmental windows.
Conclusions
Understanding the male preponderance in autism prevalence requires understanding biological processes involved in brain sexual differentiation (14, 16). Our results suggest that, on average, autistic individuals are more likely to show a biologically typical male expression of neuroanatomy. Specifically, in autistic females this shift is associated with typically sex-differential cognitive features and overlaps with sex-differential, steroid-regulated, and excitatory cell-type specific expression patterns. Identifying neuroanatomical, cognitive, and genetic interrelations at the intersection of sex-differential and autism-associated neurobiology will provide promising avenues for research into biological mechanisms underpinning the etiology of autism. Echoing recent calls for an increased inclusion of females in basic neuroscience research (120), we extend this to clinical neuroscience, where increased attention to females in neurodevelopmental research will help elucidate sex-differential likelihood factors as well as potential sex-related diagnosis and support that are informed by neurobiology.
1. : Prevalence and characteristics of autism spectrum disorder among children aged 8 years: autism and developmental disabilities monitoring network, 11 sites, United States, 2012. MMWR Surveill Summ 2018; 65:1–23Crossref, Medline, Google Scholar
2. : Sex/gender differences and autism: setting the scene for future research. J Am Acad Child Adolesc Psychiatry 2015; 54:11–24Crossref, Medline, Google Scholar
3. : What is the male-to-female ratio in autism spectrum disorder? A systematic review and meta-analysis. J Am Acad Child Adolesc Psychiatry 2017; 56:466–474Crossref, Medline, Google Scholar
4. : Editorial: sex differences in the autistic brain. Front Integr Neurosci 2021; 15:708368Crossref, Medline, Google Scholar
5. : Notice of retraction and replacement: Ecker et al, Association between the probability of autism spectrum disorder and normative sex-related phenotypic diversity in brain structure. JAMA Psychiatry. 2017;74(4):329–338. JAMA Psychiatry 2019; 76:549–550Crossref, Medline, Google Scholar
6. : Biological sex affects the neurobiology of autism. Brain 2013; 136:2799–2815Crossref, Medline, Google Scholar
7. : Testing the extreme male brain hypothesis: is autism spectrum disorder associated with a more male-typical brain? Autism Res 2021; 14:1597–1608Crossref, Medline, Google Scholar
8. : Sex differences in cortical volume and gyrification in autism. Mol Autism 2015; 6:42Crossref, Medline, Google Scholar
9. : Sex differences in frontal lobe connectivity in adults with autism spectrum conditions. Transl Psychiatry 2017; 7:e1090Crossref, Medline, Google Scholar
10. : Sex differences in autism: a resting-state fMRI investigation of functional brain connectivity in males and females. Soc Cogn Affect Neurosci 2016; 11:1002–1016Crossref, Medline, Google Scholar
11. : Default mode hypoconnectivity underlies a sex-related autism spectrum. Biol Psychiatry Cogn Neurosci Neuroimaging 2016; 1:364–371Crossref, Medline, Google Scholar
12. : Network-specific sex differentiation of intrinsic brain function in males with autism. Mol Autism 2018; 9:17Crossref, Medline, Google Scholar
13. : The early origins of autism. Child Adolesc Psychiatr Clin N Am 2017; 26:555–570Crossref, Medline, Google Scholar
14. : Convergence of sex differences and the neuroimmune system in autism spectrum disorder. Biol Psychiatry 2017; 81:402–410Crossref, Medline, Google Scholar
15. : Why are autism spectrum conditions more prevalent in males? PLoS Biol 2011; 9:e1001081Crossref, Medline, Google Scholar
16. : The extreme male brain theory of autism. Trends Cogn Sci 2002; 6:248–254Crossref, Medline, Google Scholar
17. : The extreme male brain revisited: gender coherence in adults with autism spectrum disorder. Br J Psychiatry 2012; 201:116–123Crossref, Medline, Google Scholar
18. : A phenotypic spectrum of autism is attributable to the combined effects of rare variants, polygenic risk, and sex. Nat Genet 2022; 54:1284–1292Crossref, Medline, Google Scholar
19. : The role of sex-differential biology in risk for autism spectrum disorder. Biol Sex Differ 2016; 7:58Crossref, Medline, Google Scholar
20. : The female protective effect against autism spectrum disorder. medRxiv 2021. doi:
21. : Elevated fetal steroidogenic activity in autism. Mol Psychiatry 2015; 20:369–376Crossref, Medline, Google Scholar
22. : Foetal oestrogens and autism. Mol Psychiatry 2020; 25:2970–2978Crossref, Medline, Google Scholar
23. : Linking spatial gene expression patterns to sex-specific brain structural changes on a mouse model of 16p11.2 hemideletion. Transl Psychiatry 2018; 8:109Crossref, Medline, Google Scholar
24. : Transcriptomic and cellular decoding of regional brain vulnerability to neurogenetic disorders. Nat Commun 2020; 11:3358Crossref, Medline, Google Scholar
25. : Mapping early brain development in autism. Neuron 2007; 56:399–413Crossref, Medline, Google Scholar
26. : The neuroanatomy of autism spectrum disorder: an overview of structural neuroimaging findings and their translatability to the clinical setting. Autism 2017; 21:18–28Crossref, Medline, Google Scholar
27. : Neuroimaging endophenotypes: strategies for finding genes influencing brain structure and function. Hum Brain Mapp 2007; 28:488–501Crossref, Medline, Google Scholar
28. : Brain anatomy and its relationship to behavior in adults with autism spectrum disorder: a multicenter magnetic resonance imaging study. Arch Gen Psychiatry 2012; 69:195–209Crossref, Medline, Google Scholar
29. : Interindividual differences in cortical thickness and their genomic underpinnings in autism spectrum disorder. Am J Psychiatry 2022; 179:242–254Link, Google Scholar
30. : Neurobiological correlates of change in adaptive behavior in autism. Am J Psychiatry 2022; 179:336–349Link, Google Scholar
31. : Synaptic and transcriptionally downregulated genes are associated with cortical thickness differences in autism. Mol Psychiatry 2019; 24:1053–1064Crossref, Medline, Google Scholar
32. : Towards robust and replicable sex differences in the intrinsic brain function of autism. Mol Autism 2021; 12:19Crossref, Medline, Google Scholar
33. : Image processing and quality control for the first 10,000 brain imaging datasets from UK Biobank. Neuroimage 2018; 166:400–424Crossref, Medline, Google Scholar
34. : The Autism Brain Imaging Data Exchange: towards a large-scale evaluation of the intrinsic brain architecture in autism. Mol Psychiatry 2014; 19:659–667Crossref, Medline, Google Scholar
35. : Enhancing studies of the connectome in autism using the Autism Brain Imaging Data Exchange II. Sci Data 2017; 4:170010Crossref, Medline, Google Scholar
36. : The EU-AIMS Longitudinal European Autism Project (LEAP): design and methodologies to identify and validate stratification biomarkers for autism spectrum disorders. Mol Autism 2017; 8:24Crossref, Medline, Google Scholar
37. : The EU-AIMS Longitudinal European Autism Project (LEAP): clinical characterisation. Mol Autism 2017; 8:27Crossref, Medline, Google Scholar
38. : Identification of common genetic risk variants for autism spectrum disorder. Nat Genet 2019; 51:431–444Crossref, Medline, Google Scholar
39. : Integrative functional genomic analyses implicate specific molecular pathways and circuits in autism. Cell 2013; 155:1008–1021Crossref, Medline, Google Scholar
40. : Large-scale exome sequencing study implicates both developmental and functional changes in the neurobiology of autism. Cell 2020; 180:568–584.e23Crossref, Medline, Google Scholar
41. : Genome-wide changes in lncRNA, splicing, and regional gene expression patterns in autism. Nature 2016; 540:423–427Crossref, Medline, Google Scholar
42. : Single-cell genomics identifies cell type-specific molecular changes in autism. Science 2019; 364:685–689Crossref, Medline, Google Scholar
43. : Transcriptome-wide isoform-level dysregulation in ASD, schizophrenia, and bipolar disorder. Science 2018; 362:eaat8127Crossref, Medline, Google Scholar
44. : Sex-specific impact of prenatal androgens on social brain default mode subsystems. Mol Psychiatry 2020; 25:2175–2188Crossref, Medline, Google Scholar
45. : Multimodal population brain imaging in the UK Biobank prospective epidemiological study. Nat Neurosci 2016; 19:1523–1536Crossref, Medline, Google Scholar
46. : Accurate brain age prediction with lightweight deep neural networks. Med Image Anal 2021; 68:101871Crossref, Medline, Google Scholar
47. : The ADHD-200 Consortium: a model to advance the translational potential of neuroimaging in clinical neuroscience. Front Syst Neurosci 2012; 6:62Medline, Google Scholar
48. : The NeuroIMAGE study: a prospective phenotypic, cognitive, genetic, and MRI study in children with attention-deficit/hyperactivity disorder: design and descriptives. Eur Child Adolesc Psychiatry 2015; 24:265–281Crossref, Medline, Google Scholar
49. : A survey on transfer learning. IEEE Trans Knowl Data Eng 2010; 22:1345–1359 Crossref, Google Scholar
50. : Transfusion: understanding transfer learning for medical imaging. arXiv 2019. doi:
51. : Deep net region-aligned prediction (RAP) localises life factors affecting brain aging in UK Biobank.
52. : Large-scale automated synthesis of human functional neuroimaging data. Nat Methods 2011; 8:665–670Crossref, Medline, Google Scholar
53. : NeuroVault.org: a repository for sharing unthresholded statistical maps, parcellations, and atlases of the human brain. Neuroimage 2016; 124:1242–1244Crossref, Medline, Google Scholar
54. : Intrinsic excitation-inhibition imbalance affects medial prefrontal cortex differently in autistic men versus women. Elife 2020; 9:e55684Crossref, Medline, Google Scholar
55. : Imbalanced social-communicative and restricted repetitive behavior subtypes of autism spectrum disorder exhibit different neural circuitry. Commun Biol 2021; 4:574Crossref, Medline, Google Scholar
56. : Atypical genomic cortical patterning in autism with poor early language outcome. Sci Adv 2021; 7:eabh1663Crossref, Medline, Google Scholar
57. : An anatomically comprehensive atlas of the adult human brain transcriptome. Nature 2012; 489:391–399Crossref, Medline, Google Scholar
58. : A single-cell transcriptomic atlas of human neocortical development during mid-gestation. Neuron 2019; 103:785–801.e8Crossref, Medline, Google Scholar
59. : Gene expression in human brain implicates sexually dimorphic pathways in autism spectrum disorders. Nat Commun 2016; 7:10717Crossref, Medline, Google Scholar
60. : Spatio-temporal transcriptome of the human brain. Nature 2011; 478:483–489Crossref, Medline, Google Scholar
61. : Genes and pathways regulated by androgens in human neural cells, potential candidates for the male excess in autism spectrum disorder. Biol Psychiatry 2018; 84:239–252Crossref, Medline, Google Scholar
62. : Parallel in vivo analysis of large-effect autism genes implicates cortical neurogenesis and estrogen in risk and resilience. Neuron 2021; 109:1409Crossref, Medline, Google Scholar
63. : Correlated gene expression supports synchronous activity in brain networks. Science 2015; 348:1241–1244Crossref, Medline, Google Scholar
64. : The “Reading the Mind in the Eyes” test: complete absence of typical sex difference in ∼400 men and women with autism. PLoS One 2015; 10:e0136521Crossref, Medline, Google Scholar
65. : Big data approaches to decomposing heterogeneity across the autism spectrum. Mol Psychiatry 2019; 24:1435–1450Crossref, Medline, Google Scholar
66. : The meaning of significant mean group differences for biomarker discovery. PLoS Comput Biol 2021; 17:e1009477Crossref, Medline, Google Scholar
67. : Autism attenuates sex differences in brain structure: a combined voxel-based morphometry and diffusion tensor imaging study. AJNR Am J Neuroradiol 2012; 33:83–89Crossref, Medline, Google Scholar
68. : Testing the empathizing-systemizing theory of sex differences and the extreme male brain theory of autism in half a million people. Proc Natl Acad Sci U S A 2018; 115:12152–12157Crossref, Medline, Google Scholar
69. : Attenuation of typical sex differences in 800 adults with autism vs 3,900 controls. PLoS One 2014; 9:e102251Crossref, Medline, Google Scholar
70. : Dump the “dimorphism”: comprehensive synthesis of human brain studies reveals few male-female differences beyond size. Neurosci Biobehav Rev 2021; 125:667–697Crossref, Medline, Google Scholar
71. : Incorporating sex as a biological variable in neuropsychiatric research: where are we now and where should we be? Neuropsychopharmacology 2017; 42:379–385Crossref, Medline, Google Scholar
72. : Sex beyond the genitalia: the human brain mosaic. Proc Natl Acad Sci U S A 2015; 112:15468–15473Crossref, Medline, Google Scholar
73. : Sex differences in the adult human brain: evidence from 5216 UK Biobank participants. Cereb Cortex 2018; 28:2959–2975Crossref, Medline, Google Scholar
74. : Patterns in the human brain mosaic discriminate males from females. Proc Natl Acad Sci U S A 2016; 113:E1968Crossref, Medline, Google Scholar
75. : Deep learning identifies robust gender differences in functional brain organization and their dissociable links to clinical symptoms in autism. Br J Psychiatry 2022; 13:1–8Google Scholar
76. : Imaging sex/gender and autism in the brain: etiological implications. J Neurosci Res 2017; 95:380–397Crossref, Medline, Google Scholar
77. : A meta-analysis of sex differences in human brain structure. Neurosci Biobehav Rev 2014; 39:34–50Crossref, Medline, Google Scholar
78. : Cerebral asymmetry and the effects of sex and handedness on brain structure: a voxel-based morphometric analysis of 465 normal adult human brains. Neuroimage 2001; 14:685–700Crossref, Medline, Google Scholar
79. : Sex differentiation of brain structures in autism: findings from a gray matter asymmetry study. Autism Res 2021; 14:1115–1126Crossref, Medline, Google Scholar
80. : Brain structure anomalies in autism spectrum disorder: a meta-analysis of VBM studies using anatomic likelihood estimation. Hum Brain Mapp 2012; 33:1470–1489Crossref, Medline, Google Scholar
81. : Neural networks related to dysfunctional face processing in autism spectrum disorder. Brain Struct Funct 2015; 220:2355–2371Crossref, Medline, Google Scholar
82. : The functional neural architecture of dysfunctional reward processing in autism. Neuroimage Clin 2021; 31:102700Crossref, Medline, Google Scholar
83. : Male-specific deficits in natural reward learning in a mouse model of neurodevelopmental disorders. Mol Psychiatry 2018; 23:544–555Crossref, Medline, Google Scholar
84. : Patients with autism spectrum disorders display reproducible functional connectivity alterations. Sci Transl Med 2019; 11:eaat9223Crossref, Medline, Google Scholar
85. : Atypical structural and functional motor networks in autism. Prog Brain Res 2018; 238:207–248Crossref, Medline, Google Scholar
86. : Altered connectivity between cerebellum, visual, and sensory-motor networks in autism spectrum disorder: results from the EU-AIMS Longitudinal European Autism Project. Biol Psychiatry Cogn Neurosci Neuroimaging 2019; 4:260–270Medline, Google Scholar
87. : Fetal testosterone influences sexually dimorphic gray matter in the human brain. J Neurosci 2012; 32:674–680Crossref, Medline, Google Scholar
88. : Fetal testosterone and autistic traits. Br J Psychol 2009; 100:1–22Crossref, Medline, Google Scholar
89. : Meconium androgens are correlated with ASD-related phenotypic traits in early childhood in a familial enriched risk cohort. Mol Autism 2020; 11:93Crossref, Medline, Google Scholar
90. : Maternal steroid levels and the autistic traits of the mother and infant. Mol Autism 2021; 12:51Crossref, Medline, Google Scholar
91. : Sexual dimorphism in the adolescent brain: role of testosterone and androgen receptor in global and local volumes of grey and white matter. Horm Behav 2010; 57:63–75Crossref, Medline, Google Scholar
92. : Androgens increase excitatory neurogenic potential in human brain organoids. Nature 2022; 602:112–116Crossref, Medline, Google Scholar
93. : Specification of cerebral cortical areas. Science 1988; 241:170–176Crossref, Medline, Google Scholar
94. : The development of gyrification in childhood and adolescence. Brain Cogn 2010; 72:36–45Crossref, Medline, Google Scholar
95. : Sex hormones in autism: androgens and estrogens differentially and reciprocally regulate RORA, a novel candidate gene for autism. PLoS One 2011; 6:e17116Crossref, Medline, Google Scholar
96. : Cross-disorder comparative analysis of comorbid conditions reveals novel autism candidate genes. BMC Genomics 2017; 18:315Crossref, Medline, Google Scholar
97. : Uncovering steroidopathy in women with autism: a latent class analysis. Mol Autism 2014; 5:27Crossref, Medline, Google Scholar
98. : Polycystic ovary syndrome and autism: a test of the prenatal sex steroid theory. Transl Psychiatry 2018; 8:136Crossref, Medline, Google Scholar
99. : Associations of maternal androgen-related conditions with risk of autism spectrum disorder in progeny and mediation by cardiovascular, metabolic, and fertility factors. Am J Epidemiol 2021; 190:600–610Crossref, Medline, Google Scholar
100. : Medical symptoms and conditions in autistic women. Autism 2022; 26:373–388Crossref, Medline, Google Scholar
101. : Gene regulation by gonadal hormone receptors underlies brain sex differences. Nature 2022; 606:153–159Crossref, Medline, Google Scholar
102. : The two faces of estradiol: effects on the developing brain. Neuroscientist 2009; 15:599–610Crossref, Medline, Google Scholar
103. : Estradiol and the developing brain. Physiol Rev 2008; 88:91–124Crossref, Medline, Google Scholar
104. : Model of autism: increased ratio of excitation/inhibition in key neural systems. Genes Brain Behav 2003; 2:255–267Crossref, Medline, Google Scholar
105. : Neural transcriptomic analysis of sex differences in autism spectrum disorder: current insights and future directions. Biol Psychiatry 2022; 91:53–60Crossref, Medline, Google Scholar
106. : The organizational hypothesis: reflections on the 50th anniversary of the publication of Phoenix, Goy, Gerall, and Young (1959). Horm Behav 2009; 55:561–565Crossref, Medline, Google Scholar
107. : Is there an association between prenatal testosterone and autistic traits in adolescents? Psychoneuroendocrinology 2022; 136:105623Crossref, Medline, Google Scholar
108. : Brain development in autism: early overgrowth followed by premature arrest of growth. Ment Retard Dev Disabil Res Rev 2004; 10:106–111Crossref, Medline, Google Scholar
109. : Early brain overgrowth in autism associated with an increase in cortical surface area before age 2 years. Arch Gen Psychiatry 2011; 68:467–476Crossref, Medline, Google Scholar
110. : Age-related temporal and parietal cortical thinning in autism spectrum disorders. Brain 2010; 133:3745–3754Crossref, Medline, Google Scholar
111. : Longitudinal cortical development during adolescence and young adulthood in autism spectrum disorder: increased cortical thinning but comparable surface area changes. J Am Acad Child Adolesc Psychiatry 2015; 54:464–469Crossref, Medline, Google Scholar
112. : Cortical morphological markers in children with autism: a structural magnetic resonance imaging study of thickness, area, volume, and gyrification. Mol Autism 2016; 7:11Crossref, Medline, Google Scholar
113. : Pathological priming causes developmental gene network heterochronicity in autistic subject-derived neurons. Nat Neurosci 2019; 22:243–255Crossref, Medline, Google Scholar
114. : Autism spectrum disorders: toward a gendered embodiment model. Soc Sci Med 2012; 74:1667–1674Crossref, Medline, Google Scholar
115. : Towards equitable diagnoses for autism and attention-deficit/hyperactivity disorder across sexes and genders. Curr Opin Psychiatry 2022; 35:90–100Crossref, Medline, Google Scholar
116. : Role of dopamine receptors in ADHD: a systematic meta-analysis. Mol Neurobiol 2012; 45:605–620Crossref, Medline, Google Scholar
117. : Potential hormonal mechanisms of attention-deficit/hyperactivity disorder and major depressive disorder: a new perspective. Horm Behav 2009; 55:465–479Crossref, Medline, Google Scholar
118. : Sex differences in dopamine receptors and their relevance to ADHD. Neurosci Biobehav Rev 2000; 24:137–141Crossref, Medline, Google Scholar
119. : Elevated rates of autism, other neurodevelopmental and psychiatric diagnoses, and autistic traits in transgender and gender-diverse individuals. Nat Commun 2020; 11:3959Crossref, Medline, Google Scholar
120. : Considering sex as a biological variable will require a global shift in science culture. Nat Neurosci 2021; 24:457–464Crossref, Medline, Google Scholar